top of page
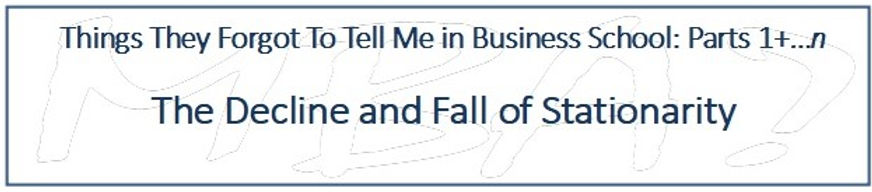
The Decline and Fall of Stationarity
Things They Forgot to Tell Me In Business School
I was saddened the other day upon reading of the passing of stationarity, my grief tempered only by my ignorance. Still, I had a moment.
And in this, I may or may not stand alone. As it turns out, stationarity is not only the term for something we all implicitly understand but may not know the name of, but it sits unassumingly at the very intersection of scientific man’s understanding of nature and nature itself. Heady stuff for a statistical construct.
Those smarter than me will already know that stationarity simply means “having fixed position”. In the context of statistical or econometric analysis, a stationary time series is one where statistical properties such as mean, variance, autocorrelation, etc. do not change over time. Or, in plain English, “a stationary process is a stochastic process whose unconditional joint probability does not change when shifted in time” (Wikipedia). Really. The crux is that data in stationary time series is not static, it is just that the manner in which it changes does not change, which makes it invaluable for forecasting.
Stationary data is a cornerstone of statistical forecasting, trend estimation, and causal inference. Without stationarity, you do not have the constant slope that [theoretically] allows you to load up a crate of assumptions and look backward in order to make a great leap forward. Stationarity is as much an article of faith as a statistical state, born of necessity and convenience [see efficient market hypothesis for another example, same same but different].
Such is its utility that if your data isn’t conveniently stationary on its own, displaying say a trending mean, it can be transformed, de-trended, or otherwise “stationarized” until it is. Now a bystander may zero in on the strangeness of adjusting your feet to fit your shoes, but those with more expertise insist on there being only one size shoe in the rack.
To be more candid this was not a blanket “deceasing” of stationarity, or even a particularly recent or universally accepted one, but rather a targeted lament connected to climate change, in particular, a surge in severe storm events as part of the global water cycle going haywire. The gist being that accelerating changes in the earth’s climate means that past conditions are increasingly unable to be reliably used to forecast possible future states. [Please note that no other presumed stationary data, in the biological sciences or elsewhere, were harmed in the making of this accusation].
And even in climate sciences, stationarity does not break like a dry stick, but rather confidence in it erodes over time as people first try to account for increasing anomalies in recent years before ultimately giving up “calculating the odds” and resorting to tactics from nascent fields like Decision Making Under Uncertainty, which could be known as Deep Guessing, or more established ones like Competitive Decision Making, which is known as Game Theory - but do so only at last resort.
This situation gets in the way not only of progress but of continuity, which is even worse. And not only in a progressive “triple bottom line” kind of way, but by wicking its way up through the roots of more conservative, if infinitely darker, arts such as commercial risk assessment, actuarial sciences, as well as civil engineering and industrial design, just to name a few.
The importance of stationarity to the insurance and banking sectors, where risk is assessed and priced against probabilities, can scarcely be exaggerated. And, like so much else in business and life, it is a confidence game. Few at the pointy edge of commercial affairs are in the business of calculating key underlying climate-related risks, they consult tables, charts, or maps prepared by others, often government ministries or large underwriters before plugging results into proprietary models providing answers tailored to their needs. And all goes well as long as they maintain confidence in these outside inputs.
Once an insurer loses confidence in the predictive value of existing data on, say, lightning strikes involving humans due to major storm events, it becomes impossible to price and market personal lightning strike risk insurance to the tinfoil hat crowd. And this will be the same for more serious examples. Likewise, if lenders are no longer able to confidently calculate storm and flood risk based on past environmental data this makes many types of lending riskier and significantly more expensive or untenable, particularly but not exclusively against fixed physical assets, transport and logistics.
This also falls near the heart of my business of city planning and development where I spend a lot of time on urban design considerations and infrastructure specifications, forecasting the needs of urban populations in 5, 10, or even 100 years in the future for everything from schools and parks to potable water systems, flood control, electricity, and transportation networks. Erratic and severe climatic conditions inject another costly level of uncertainty into a speculative process where progressiveness is already tempered by deep caution due to high initial costs, large operating costs, and even higher retrofitting costs if you get it really wrong.
My own targeted lament is that while climate change denial has proven untenable, people now fall all over themselves ideologically about causality and responsibility when increasingly there are facts on the ground that need addressing. After all, once the tide is cresting in your living room it becomes less material than what brought it there, and far more important to figure out what to do about it.
bottom of page